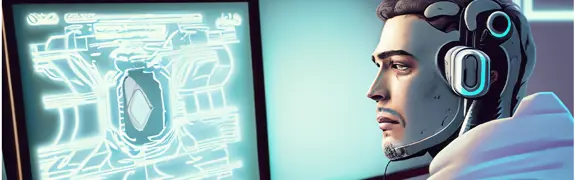
using AI to build software and how to avoid the race to the average
Using AI to build software can result in a slippery slope towards developing average code.
PLEASE ACCEPT OUR. COOKIE POLICY
There are many uses for Redis Cache but one standout use is for websites that display the results of complicated numeric models. This is very common in the Financial Services and Insurance industries.
Often, numeric models require the loading of a lot of information, very often from databases. Then that information is used to create a lot of calculations within the model and eventually, the end result(s) are produced.
In these situations storing the numeric models in Redis Cache in Azure delivers huge benefits, and the larger the model, the larger the benefits.
Imagine the following scenario, which is very common in financial services and insurance:
User 1 enters a website, calls a webpage, data is loaded from a database. There's a lot of data so that takes 5 seconds. Calculations are then done on the data. There are a lot of calculations so that takes another 10 seconds. The results data is then loaded to the webpage and there's not much of it so it only takes few milli-seconds more. In total it takes 15 seconds and a few milliseconds for data to be displayed on the page.
User 2 enters the website, calls the same webpage as user 1, data is loaded from a database, calculations are done on that data, and the data is loaded to the webpage. It takes 15 seconds and a few milli-seconds for data to be displayed on the page.
User 3 enters the website, calls the same webpage, data is loaded from a database, calculations are done on that data, the data is loaded to the webpage. It takes 15 seconds and a few milli-seconds for data to be displayed on the page.
Do you see any wastage in the above scenario?
Using Redis Cache in Azure however, users can load the end models straight away without loading data from the database or processing the calculations. The loading of the data from the database and the processing of the model results could have been done either overnight or when User 1 called the webpage. Once the model is first calculated, the results can be stored as a complete object in Redis Cache.
Creating a Redis Cache in Azure is extremely simple.
To demonstrate the benefits, I've prepared a brief demonstration project on GitHub. It also has a database creation script. When you load the project you can change the number of models and the number of inputs in each model.
Using 10 models and 10 variables per model, you can see that the first time you run it, loading the data and creating the models takes 10 seconds. Loading the models from the cache takes a few milli-seconds.
The second time you run, if you keep the number of models and the number of inputs the same then there is no processing to be done and the results are simply loaded from the cache. Therefore it only takes a few milli-seconds to load the results.
Think of how many situations this could apply to in your company, and how much it could speed up some of your slow sites.
You don't have to using Azure for anything else in order to use Redis Cache in production. You can run all your existing infrastructure on premises and then just have the results loaded from Redis Cache in Azure.
Give it a try.
Using AI to build software can result in a slippery slope towards developing average code.
Using DotNet 8 and Aspire With Azure Container Apps Makes Kubernetes Enabled Apps Incredibly Easy
Moving to a micro-services architecture can sometimes seem daunting due to the amount of technical choices to be made and the amount of planning involved. Often however, the migration can be made in manage-able steps. Here is one classic example.
Fast Endpoints have become very popular with .Net developers recently, but how much faster are they than regular Controller endpoints using .Net 8
GPT4 and other tools have good text summarisation capabilities but only Deep Mind is providing the real breakthroughs.
Managing Infrastructure State can be tricky in the cloud. AZTFY and Terraform can help with management